MLCommons Releases New AI Benchmark Tests for Speed and Efficiency
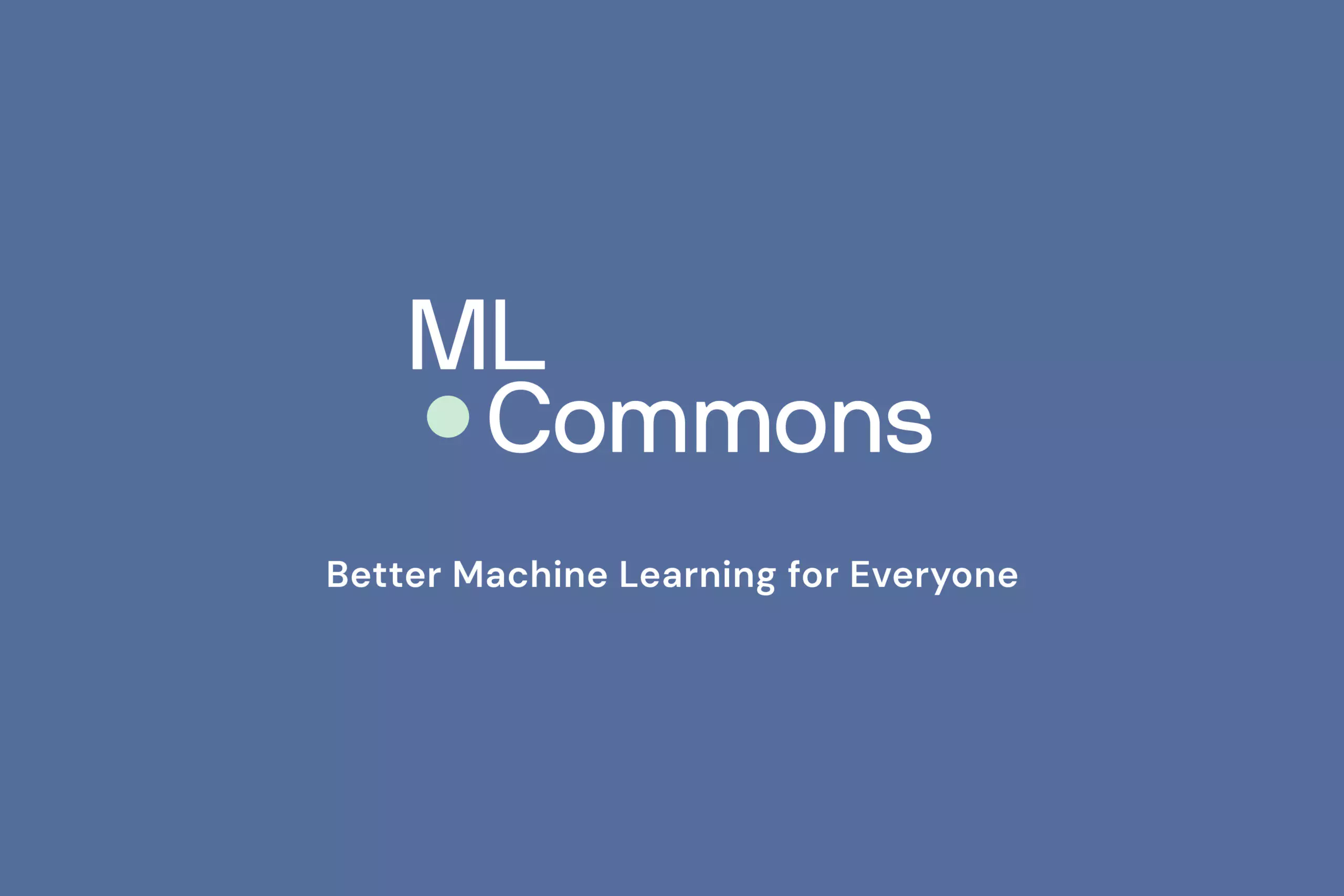
MLCommons Introduces New AI Benchmarks for Speedy User Query Responses and Text-to-Image Outputs
MLCommons, an AI benchmarking group, has released new benchmarks focusing on the speed and efficiency of AI applications. Learn how these benchmarks measure the speed of responses for AI models like ChatGPT and text-to-image generation.
MLCommons Releases New AI:Learn how these benchmarks measure the speed of responses for AI models like ChatGPT and text-to-image generation.
MLCommons, a renowned artificial intelligence benchmarking group, has unveiled a new set of benchmarks aimed at evaluating the speed and efficiency of AI applications. These benchmarks, released recently, specifically assess how quickly high-performance hardware can execute AI applications and respond to user queries.
The latest benchmarks added by MLCommons focus on measuring the speed at which AI chips and systems can process and generate responses from data-rich AI models. These results provide valuable insights into the responsiveness of AI applications, such as ChatGPT, in delivering timely responses to user queries.
One of the newly introduced benchmarks, Llama 2, is designed to measure the speed of question-and-answer scenarios for large language models. Developed by Meta Platforms, Llama 2 boasts an impressive 70 billion parameters, showcasing the capabilities of modern AI systems.
Additionally, MLCommons has expanded its suite of benchmarking tools with a new text-to-image generator benchmark, based on Stability AI’s Stable Diffusion XL model. This benchmark evaluates the efficiency of AI systems in generating image outputs from text inputs.
Notably, servers equipped with Nvidia’s H100 chips, including those from Google, Supermicro, and Nvidia itself, demonstrated superior performance in both new benchmarks. However, server designs featuring Nvidia’s L40S chip also showed competitive results.
Krai, a server builder, showcased a design for the image generation benchmark that utilizes a Qualcomm AI chip, known for its energy efficiency compared to Nvidia’s high-performance processors. Intel also submitted a design based on its Gaudi2 accelerator chips, which delivered promising results in terms of performance and energy efficiency.
While raw performance remains a crucial metric for AI applications, energy consumption is equally important. Balancing performance with energy efficiency is a significant challenge for AI companies, and MLCommons’ benchmarks aim to address these concerns by providing insights into power consumption alongside performance metrics.
Share this post :
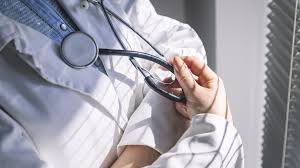
Thane Professor Sentenced to 3 Years for Harassing MBBS Trainees
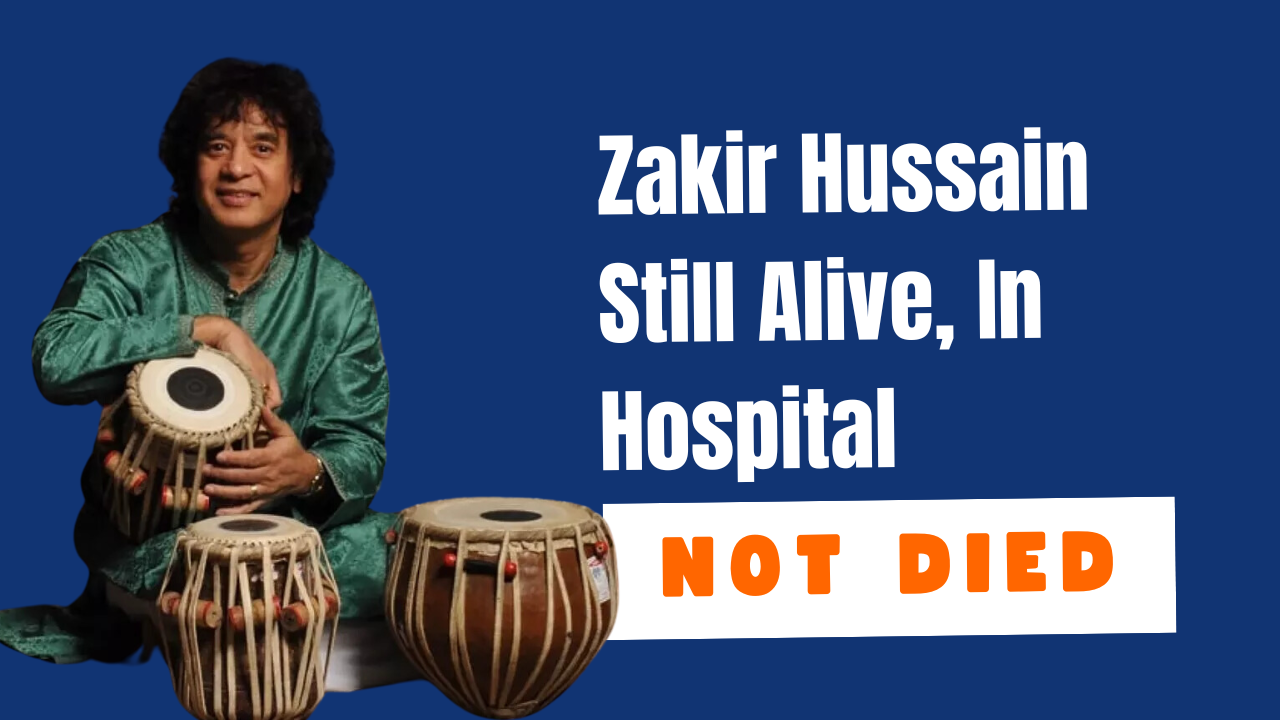
Zakir Hussain Family Requests Prayers and Clarifies False Death Reports
KTR Accuses Revanth Reddy’s Brother-in-Law of Securing Lucrative Government Contracts with Minimal Business Background
NASA says: Two Big Space Rocks To Dangerously Flyby Earth Today
Subscribe our newsletter
Purus ut praesent facilisi dictumst sollicitudin cubilia ridiculus.